Eva-Valérie Gfrerer on Using Artificial Intelligence as a Barometer for Confident Decision Making
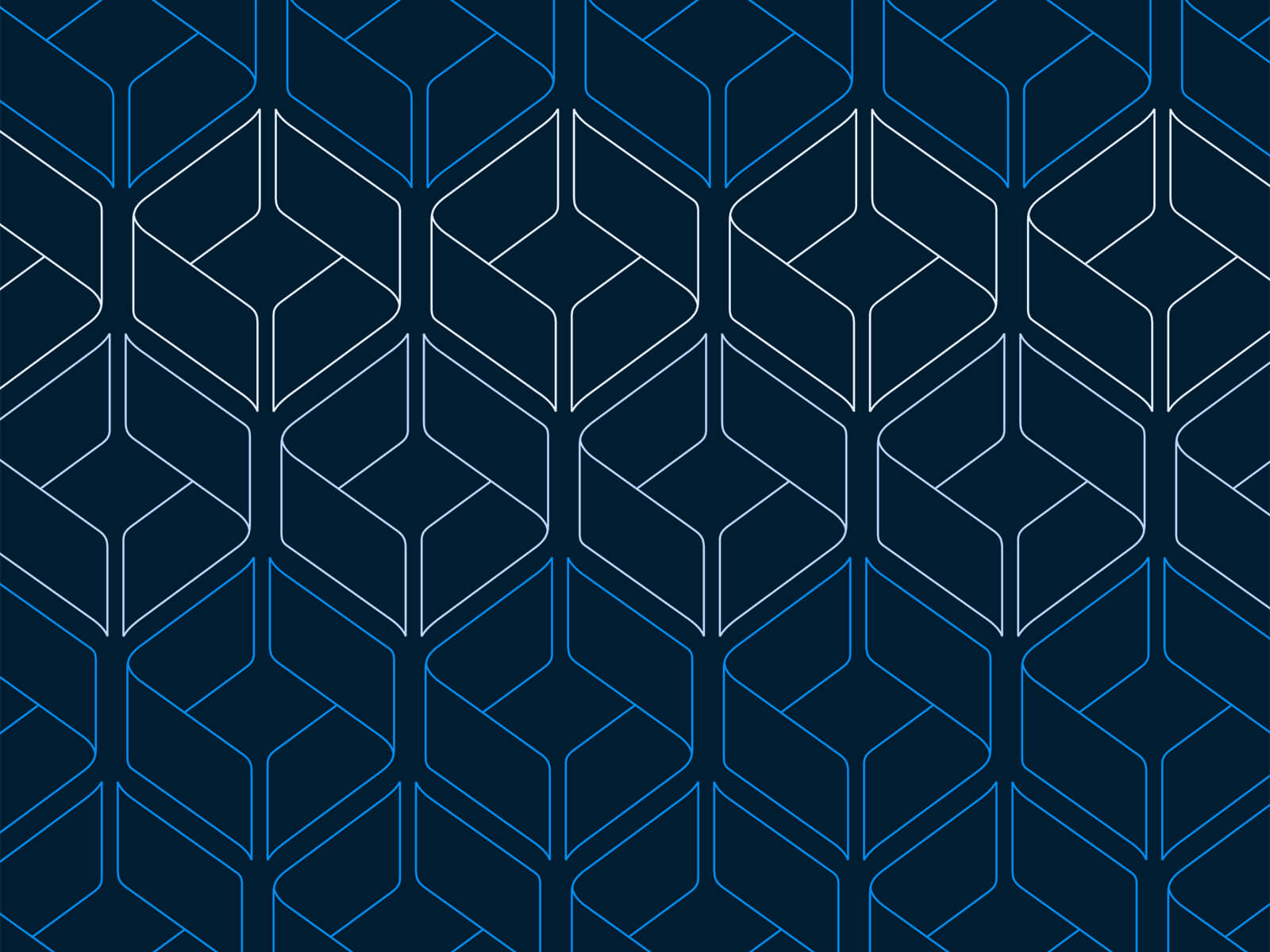
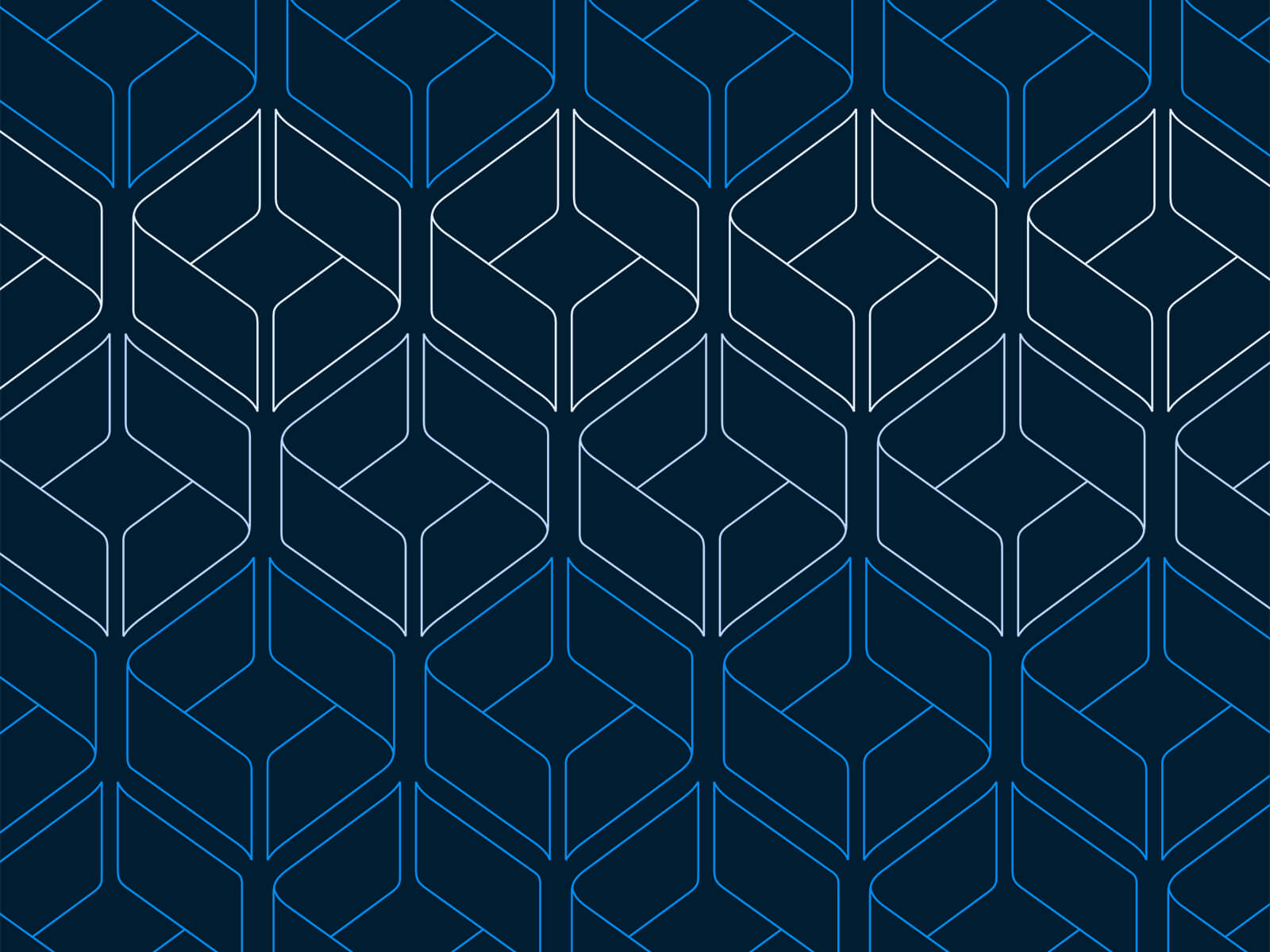
Venture capital is the engine of the startup industry and the lifeblood of innovation. Historically, the decision for who gets funding and what technology and projects will shape our society has been decided by a small and exclusive pool of homogeneous people. These decisions may impact how people around the world live, communicate, and work.
The decision-making process hasn’t evolved much technologically over the past few decades. It’s still very analog, which opens it up to the individuals’ subjective biases in the organizations. Venture capital is responsible for some of the world’s most significant innovations– ironically, it hasn’t experienced much internal innovation.
Many reports point to the venture capital industry being broken for its lack of diversity and inclusivity, but the dysfunction is much more profound.
Eva-Valérie Gfrerer, CEO & Co-Founder at MorphAIs, joins the Kauffman Journal to describe how her and her team’s innovations can solve the inefficiencies of the venture capital industry. Eva-Valérie is a behavioral scientist determined to refine our understanding of human behavior at scale with data. Along with Co-founder and machine learning scientist Jan Saputra Mueller, the company is now joined by a team with diverse scientific and other academic backgrounds in behavioral science, mathematics, statistics, sociology, and economics.
How did the idea for MorphAIs form?
84% of early-stage funds in the United States that delivered less than the minimum expected returns to their investors might be making the same mistake or mistakes.
Prior to formally starting MorphAIs, we interviewed two dozen venture capital firms in Europe and the United States about their deal-flow, sourcing, and due diligence. Unsurprisingly, most of the firms we interviewed were very heavy on human desk research, like using Google and Crunchbase. This manual process is prone to certain unconscious biases, which promote unconscious discrimination against people outside of a typical entrepreneurial profile.
People tend to seek commonalities with other people to build that critical level of trust that precedes investing capital. Since most VCs have a typical profile, this bias makes it more difficult for women and people of different ethnicities to raise capital.
Rather than waiting for the industry to become more diverse to hopefully counteract the current biases, we started MorphAIs to use cold, objective data to understand why funds are underperforming.
I believe that this technology is scalable, unlike a system that is dependent on a human decision-maker.
From Structured Data to AI-based Recommendations
People use personal judgment as their primary means of making decisions when only limited information is available.
When structured data enters the equation, we can incorporate more data points into our interpretation. While this decreases some level of subjectivity, the process is still susceptible to personal bias.
Suppose I look up the founder of a company I’m considering investing in on LinkedIn: I see he went to NYU, and immediately a particular judgment happens: Oh, my sister went there. She’s a great person and really smart. I think this school is very good, and this is probably a good founder because he went there. Similarly, if I see a school I don’t recognize, I can think the opposite.
Of course, investors do more due diligence than this, but that’s just one example of an entry point for human bias to cloud the decision-making process.
Decision-making will be inefficient as long as humans must shoulder the full responsibility of interpreting every data point.
Going from structured data to AI-based recommendations allows a much wider array of data to be analyzed and interpreted. For example, an algorithm can create a university score that encapsulates the number of successful founders that went to a particular university and generate some sort of variable for how impactful a certain school is on success.
Now, add millions of other data points to find functionally useful variables. For example, how about a network score for individuals that come from a university? Perhaps it isn’t the university education that is the dominant success factor, but the strength of the alumni network of this university. Now, this may be a bias as well but it gives a notion of how we aim at identifying the root cause of success factors.
Artificial intelligence allows us to see patterns far outside the reasonable realm of human analysis with speed and precision. We can refine individual variables of success by analyzing millions of data points and then assigning certain weights to each variable to predict success.
We want to build the technology that can understand and predict early-stage startup success. This can come in any number of ways that produce distinct operational advantages. For example, our model could help produce a deal flow beyond a firm’s individual network and expose talent that might not be as visible. We can accomplish this while getting a more clear perspective of what actually catalyzes success in venture capital.
Deductive models would say white men are more successful in founding companies, and we don’t think this is accurate or functionally capable of predicting future success.
Recent studies point to women-led or minority-led teams as providing significantly higher returns than homogenous teams. We can induce evidence from studies into our models to better predict actual success. While for example, women-led or minority-led teams would not show up as more successful simply based on data analysis because the database itself is biased, we can induce this knowledge in our models to make fairer investment decisions.
Well, If the Data Itself is Biased, Wouldn’t AI Extrapolate on Those Biases?
There’s a challenge with using artificial intelligence in venture capital because it seeks to find patterns in existing data sets.
So, if our thesis for this conversation is that artificial intelligence can be used to help investors find incredible opportunities coming from underserved markets, wouldn’t the notion that artificial intelligence algorithms will just spit out more of the same?
The answer is, that it depends.
If we use standard Machine Learning techniques that are purely based on observational data and don’t integrate additional human knowledge about our world, we will definitely reproduce bias. That has to do with the fact that those methods are purely deductive.
On the other hand, if we use Bayesian inference, we can also include explicit knowledge and assumptions about the world, such as symmetry assumptions. One plausible assumption could be that the ability to found a successful startup is independent of gender. This kind of knowledge can be encoded mathematically and used in our models in addition to purely observational data, which allows our model to use inductive reasoning in addition to deductive reasoning. In the end, this allows us to eliminate bias.
We can use artificial intelligence to differentiate between deductive and inductive reasoning. Deductive is what’s coming from the data, and inductive is when assumptions enter the picture. We can effectively vary the degrees of inductive reasoning into the algorithm by changing the weighting of certain factors based on assumptions.
AI technology isn’t inherently biased, but if it’s trained on discriminatory data, then it might also make biased suggestions.
There are other professions incorporating AI that have a much more difficult challenge than venture capital. For example, AI-based human resources can be challenging as the optimal result is usually defined by subjective interpretation. In other words, the data points in and of themselves may be flawed. It’s much easier to analyze the success or failure of a startup– you have apparent output variables.
We want to use those output variables to reverse-engineer the ingredients to build a technology-enabled means to make better decisions. The removal of bais is just a by-product.
How Can You Remove Bias?
We analyzed over 1 million companies over the past 20 years in the startup scenes in Europe, Israel, Canada, and the United States over a hundred industries and identified 150 success indicators.
So, to explore whether diverse-teams will achieve higher-returns, we used our algorithm to compare a group of female CEOs to a group of male CEOs. The data showed that despite it being more difficult for female CEOs to get funding, they actually did outperform the male CEOs once they do.
Some data points are so few that they pale in comparison to twenty years of history, but this shouldn’t disqualify them. We believe that they hint at a much more significant trend in venture capital and should be explored.
Still, the technology assumes that because the vast majority of the companies in the past were male-founded, male-led, or male-invested, a company today with the same makeup will be more successful than not.
Purely based on historical data, male teams might seem to be more successful because the data pertaining to the whole process of bringing a startup to success is biased.
So, in theory, female teams may find more difficulty raising capital in a world where most decision makers are white males. This perpetuates a cycle of less successful female teams leading to fewer data points that support the notion that female teams can be successful.
However, it’s impossible to draw conclusions simply from deductive observations, especially when the data is biased. By combining data driven methods and making plausible assumptions with inductive reasoning, we can make superior investment decisions.
How Can Artificial Intelligence Improve the Overall Decision Making Process?
Technology is more than just a means to decrease bias in the screening process; it can help magnify the human elements that significantly contribute to the process and accelerate human change.
Data illuminates which variables are most closely related to startup success, which allows for more accurate investments and fewer mistakes.
40% of VC time is spent on screening and sourcing, and only around 30% is spent on building the team and helping portfolio companies.
Now, suppose a technology-driven process is able to partially or fully automate the screening and sourcing process, allowing firms to analyze more companies in less time than their human resources. A technology-driven sourcing and screening strategy will allow venture capital firms to analyze thousands of companies with digital footprints at a fraction of the time of human analysis.
Imagine not having to spend 40% of your time sourcing and screening. Instead, you could allocate that time to adding value to your portfolio companies.
MorphAIs wants to build a hybrid model that VCs can leverage to do the bulk of the heavy lifting so that they can make the best decisions with the most qualified pieces of data.
Artificial Intelligence as a Barometer for Confident Decision Making
The application of artificial intelligence to venture capital is still very new in practice, and there is much ground to cover.
The lowest hanging fruit for artificial intelligence is to create obstacles to overconfidence.
For example, 70% of car drivers think they’re better drives than everyone else on the road.
96% of VCs believe that they make better decisions than the rest of VCs.
Mathematically, the latter scenario is more preposterous than the first.
Experience is a very important factor to investor success and gut-feeling is a translation of experience as a heuristic. We want to build a system that works with intuition rather than against it.
Gut feeling comes from experience because you, as a great professional and have developed personal heuristics that make it easier and quicker for you to make personal decisions.
The problem is that very often your heuristics don’t adjust to new situations or circumstances as quickly over time. It’s difficult to adjust certain trained routines, and the lack of flexibility can be detrimental to those in venture capital.
Some static variables may have a continuously strong impact over time, but dynamic variables like industry trends and business models may change in influence over time.
It’s natural for people to overestimate their capabilities. Although overconfidence may serve a very useful purpose in an environment like venture capital where everything is uncertain, it can still have a negative impact on decision accuracy.
We view artificial intelligence as a means to emphasize the best elements of confidence while minimizing the negative. Technology has the potential to train and objectivize decision-making. It can help you better slow down when you’re running too fast, or sprint when you’re too slow.
For example, if we’re able to look at cold hard metrics void of emotion, we may think twice about some decisions we rush into with bravado or give us pause to re-evaluate decisions for which we don’t feel as strongly.
Picking the right company at an early stage generally yields the highest returns. Since this phase is significantly more risky and ambiguous than later stages, humans are pushed to make more snap judgments or over-dependence on ingrained heuristics than usual, which is where the bulk of biases occur and discrimination happens.
Greater accuracy in the early stages trickles into success in the later stages.
VCs can be fairly technologically averse, and we don’t want to wait for the gradual adoption of better decision-making.
The best way to actually invent something is to build it and use it yourself rather than waiting for the market to adopt the technology. We’re confident that this technology will help change the industry, so we’re raising our own fund this year to be the agents of change ourselves. I believe the technology can make a difference, and once we’ve proven our success with it, we can open it up for integration to external funds.
There are many challenges ahead for the reinvention of the venture capital industry. We believe we can leverage artificial intelligence to test what works and what doesn’t, either in real-time or simulation scenarios, at a much faster rate than the current pace of innovation.
The role of investors must evolve from being a gatekeeper to what may be the solutions to some of the world’s most enormous problems, and we’re confident that artificial intelligence can take us there.
Originally published at https://www.kauffmanfellows.org on March 4, 2021.